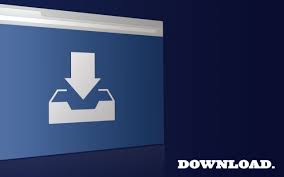
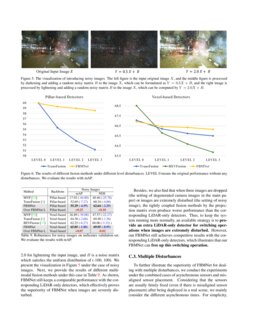
GT represents the optimal performance that an algorithm is desired to achieve. The model’s performance improves as the amount of annotated data increases.These annotated data are known as Ground-Truth (GT) and used for training, testing, and evaluating the models. However, DL- and ML-based applications usually demand a large amount of annotated data to train the model, which relies on human experts with specialized knowledge and clinical experience to achieve adequate results. It also helps to predict the number of patients of upcoming days in pandemics. The ML and DL methods help doctors diagnose the disease, predict its risk, and prevent them at an appropriate time. In recent years, medical image analysis has been boosted by ML and DL. It utilizes hierarchical recombination of features to extract relevant information and then learn the pattern representation by employing a multi-layer neural network. DL models have achieved massive progress in terms of algorithms, applications, and theories.
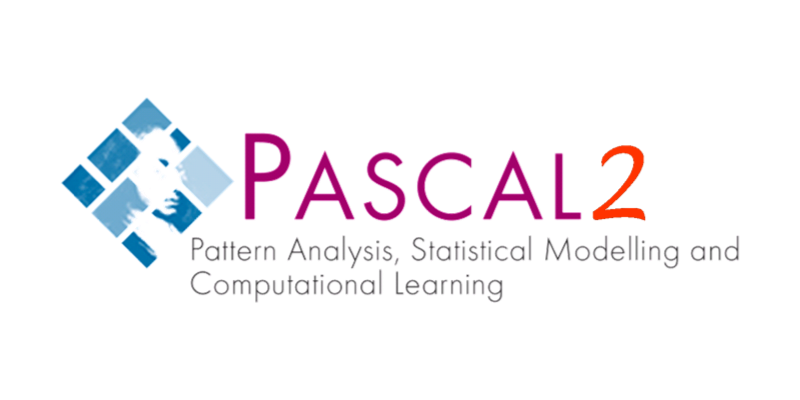
ĭeep learning (DL) is a subset of ML, which aims at learning many levels of distributed representations of the data to be modeled. ML has been applied to different fields including robotics, pattern recognition, data mining, object recognition, face detection, and medical diagnosis. ML is a subfield of artificial intelligence (AI) which includes a wide range of computational algorithms and modeling tools utilized to process large numbers of data, where these algorithms aim to mimic human intelligence by learning from training data. The relatively recent development of very powerful computational hardware, such as graphic processioning units (GPUs), and the development of deep neural networks, paired with the availability of large quantities of digital data, have facilitated for machine learning (ML) to emerge as a field with the potential to generate great progress in different fields. The main contribution of this study is to provide an intensive review of the popular annotation tools and show their successful usage in annotating medical imaging dataset to guide researchers in this area. In this survey, we present the currently available annotation tools for medical imaging, including descriptions of graphical user interfaces (GUI) and supporting instruments. Different annotation tools have been developed to assist with the annotation process. Despite the huge success of deep learning algorithms in image analysis, training algorithms to reach human-level performance in these tasks depends on the availability of large amounts of high-quality training data, including high-quality annotations to serve as ground-truth.
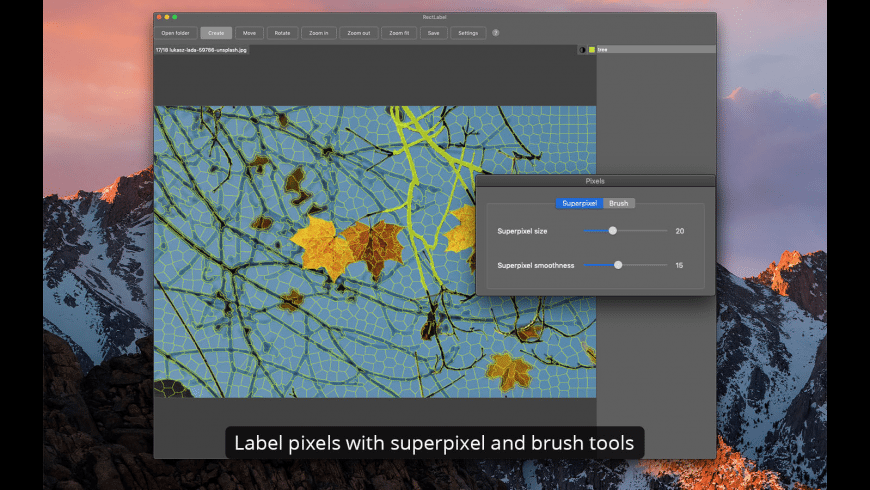
Deep learning and machine learning techniques provide different solutions for medical image interpretation including those associated with detection and diagnosis. It requires significant expertise to efficiently and correctly interpret the images generated by each of these technologies, which among others include radiography, ultrasound, and magnetic resonance imaging. fastai/fastai/blob/2d399f7cbca604964329cb38fc68569ba32af27a/courses/dl1/fish.Medical imaging refers to several different technologies that are used to view the human body to diagnose, monitor, or treat medical conditions. Just found this as well, did a search bbox in the new repo and found this as well. Hope that helps, sometimes (often?) it seems like figuring out what goes in and what goes out is the hardest part… 95% time spent preparing the data, 5% training I have a function to translate either labelImg (xmls) or RectLabel (jsons) annotations to that pickle format if you want. You can feed that pickle and the path to your images to the SSD. 1.) for example.īut remember img1_bbox_1 is a flattened list. but if you have 3 classes it will be two values e.g (1. If you have 2 classes, then it’ll be one value only one_hot_encoding = 0. And one_hot_encoding is … well the one hot encoding. With (x_bl, y_bl) being the coordinates of the bottom left point. Here img_bbox is itself a vector containing 4 + C-1 values with C the number of classes. Nothing very hard here, just needs to understand that they save a dict as pickle in that following scheme Turn these annotations into the proper format expected by the SSD implementation.
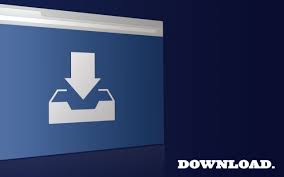